Editor’s note: This case study was originally published in 2020. It has been updated for accuracy and to reflect modern practices.
Here’s a secret: In the world of eCommerce PPC, maximizing return on ad spend (ROAS) does not always maximize profitability.
Depending on your product margins, focusing solely on ROAS could actually prevent your Google Ads accounts from achieving impressive growth.
If you want to discover real opportunities for profit maximization, you’ll have to look at some other data points instead.
In the following eCommerce data analysis case study, we’ll explain how connecting the data between Google Analytics, Google Ads, and unique business stats resulted in 72% more transactions at an 8% increase in spend, year over year, for one of our clients.
Along the way, we’ll also share some recommendations to help you do the same — and uncover new opportunities for improving your accounts’ performance.
ROAS: Not Always the One True Source of Power
For many eCommerce businesses, ROAS is a great measure of profitability. And, when products share the same margin, optimizing for this metric is pretty simple.
But, when multiple products have different margins, things can get a bit murkier — and require a more nuanced data analysis.
Google Ads (formerly known as Adwords) offers many bid optimization levers to eCommerce companies, such as device type, audiences, geography, and more. Add in the standard KPIs associated with pay-per-click advertising — costs, clicks, conversions — and you have a plethora of data points to consider in your “profitability” equation.
If you can correlate all of these together toward profit in your campaigns, you’ve taken your optimization abilities to the next level.
By targeting your best-performing products and layering in as many of these key metrics (“segments”) as possible, you can find profit that a focus on ROAS alone wouldn’t deliver.
Client Background
Before starting any new PPC campaigns with a client, we focus on understanding their business as much as possible: past and current marketing efforts, performance, and product potential.
For this particular client, we started by becoming familiar with the constantly fluctuating profit margins of the approximately 600 products on their site — no small feat!
Once we did that, it was all about incorporating that data directly into their marketing campaigns for better results.
The Challenge
With performance data changing so frequently and products going in and out of stock during highly seasonal shopping periods, calculating what ratio we needed to be profitable (or simply break even) was crucial — but complicated.
Every transaction on this client’s site could theoretically have a different net profit number. To understand the exact profit line for each product, we needed to track:
- Campaign
- Ad group
- Keyword
- Search query
- Device
- And any other segments traditionally used to optimize targeting
Ideally, these data points would also help us answer some crucial questions:
- What campaigns do people come to the website from?
- What do they end up purchasing?
- What profit did the purchase generate?
- How does that profit data correlate to key targeting segments (such as search term, device, audience segment, demographic segment, etc.)?
In other words, based on the final purchase each user made, what was the profit from the final transaction and how could we distribute that profit across our PPC segments?
The Solution
Here’s what our PPC experts did:
Understanding ROAS & ROI
Before we could dive into these data points in more detail, we needed to understand the relationship between the client’s campaign ROAS and return on investment — knowing, as we did, that profit does not always equal ROAS.
To separate our ROI from our ROAS, we used the following equations:
- ROI = Profit / Ad Cost
- ROAS = Conversion Value / Ad Cost
When testing those formulas on a campaign level, the two were clearly independent figures, as seen in the example below:
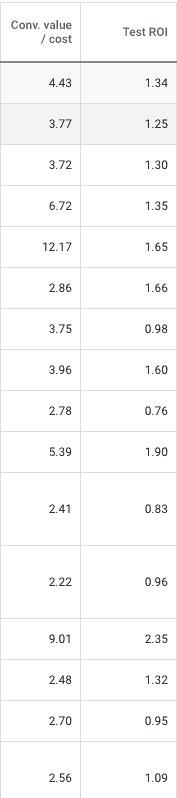
Because the test campaign ROI varied so greatly, we confirmed that campaign ROAS was not correlating with profitability.
However, doing this type of analysis on an ongoing basis proved difficult. There were data processing capability restrictions and inconsistencies in how different analytics platforms reported results, which meant we had to cross-check every data point several times.
So, we needed another approach to efficiently (and accurately) track this campaign data.
Analytics Auto-Tagging
We started with a customized analytics report (outside of Google Analytics) to pair transaction IDs with margin and profit, segmenting by ad group, keywords, device, and more.
While this got us granular product profitability data for our Google Ads campaigns, it took way too much time to import and analyze the data manually.
Because we were dealing with big data analytics in real-time, the best solution for live ad campaign optimization was going to be automation.
Our PPC team was already comfortable combining backend profitability data with each transaction. The only missing piece was connecting those transactions with a GCLID.
Once we associated GCLID tracking with transactions, we could use the data-import feature to create a new conversion for profit — and add that attribution data directly into Google Ads.
Remember: To create a custom ROI metric in Google Ads, configure the conversion so that it shows up in the “All conversions” report, not the normal conversions report. This allows for more flexibility in measuring profitability within those reports that prevent you from using a conversion-type segment.
With the time-consuming work of manually importing and confirming the data now automated, we were able to focus our work more closely on our PPC data analysis and subsequent campaign optimization.
We no longer had to build custom reports for segmented data; we could instead rely on the standard reports within Google Ads, because profit data was now baked into those. We could even look into additional segments, such as geography, time of day, target audiences, demographics, and more!
The Results
Now that we had collected enough data, we could easily optimize our PPC ads for profitability by keyword, device, demographic, and audience bids, rather than just chasing ROAS. And, with the live ROI data attached to our PPC reports, we could be more nimble (and confident) in our campaign decisions.
Because we linked all this data between Google Ads and the client’s backend, we could evaluate results on the fly as any shifts in product profit margins happened — moving more quickly to decrease cost per click (CPC) within ineffective segments.
The results: More cost-effective performance across the account, including more transactions and more profit for our client’s most popular products.
Campaign Example: Seasonal Products
From experience, we know that eCommerce seasonality is an important factor in paid search advertising. With the time we got back from our automated reporting, we could take a closer look at our seasonality data — and create some new strategies for improved profitability.
Even though ROAS data of the past isn’t necessarily indicative of future profitability, we can still use it as a directional indicator. In this case, we looked at the past two years of campaign performance to identify which products showed an increase in efficiency (ROAS) and revenue/transaction volume. This would indicate whether they had maximized traffic in the past, giving us a starting point for our current campaigns.
The difference this year? We had the actual profitability data to be more confident in our forecasting, optimizations, and investments.
After comparing the pre-holiday and holiday seasons, we isolated 30–40 products that demonstrated a significant increase in performance and conversion rates. Our strategy: Group them together to bid more aggressively, leading to more traffic, more conversions, and a higher ROI.
After implementing our new tracking in mid-July, we focused on collecting data and optimizing bids through October to prep for the upcoming holiday season. Thanks to this PPC data analysis, we started to see transactions significantly outpace the previous year starting around November, carrying us into a strong holiday shopping season.

While costs did increase slightly, the increase in transactions in December alone far outpaced them, with a 72% increase in transactions and a 68% increase in revenue year over year.

Any time that you can increase both spend and ROAS, it’s a huge win. It’s even better when you can prove the profit margin (ROI) is increasing, too.
In closely tracking cost (blue) vs ROI (red) for this client, anything over 100% was pure profit. And, in December 2019, our calculated ROI outpaced cost for the first time, as seen in the screenshot below.
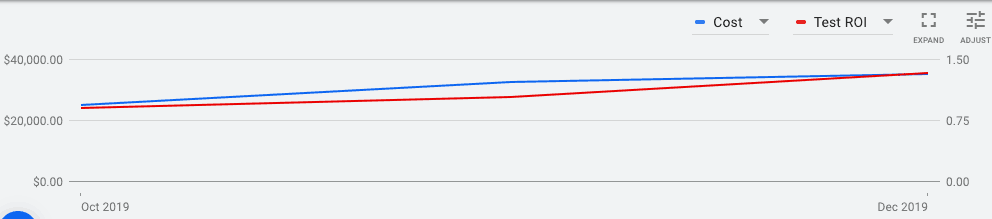
Thanks to our PPC analysis and optimization, the client not only saw costs increase but also higher transaction volume, increased ROAS, and improved ROI: a win-win scenario.
You don’t always see immediate results from data-driven optimization, but it’s often pretty clear to see exactly when your efforts are rewarded. In this case, our lift in results correlated closely with our heavy lifting on the optimization front — proving that our PPC campaign analysis was working.
How Your Business Can Replicate This Strategy
A data-driven PPC approach requires multiple layers of data collection and analysis. It won’t always be easy, and it may require some creativity with automation, but it often makes a huge difference in your bottom line.
The more in-depth knowledge you have of your eCommerce business and products, the easier it will be to emulate our team’s strategy.
Remember: While instant results from PPC do happen, they’re not necessarily the norm. Collecting this data set is crucial, but the analysis process is not “plug-and-play.” You’ll need to incorporate your own business knowledge and historical ad performance to determine the best approach for your marketing team.
Key to that is understanding that ROAS does not always equal profitability. To best increase your profit margins, you’ll need to incorporate other important business metrics with a strong understanding of eCommerce Google Ads strategies and tracking. When you do, good decisions will follow.
If it all sounds too complex for your team, we recommend working with a digital marketing agency like Inflow. Our PPC experts are trained in this kind of analysis, which means they can quickly (and efficiently) discover the best search engine marketing strategy for your brand based on historical data and their knowledge of analytics tracking.
Get started by requesting a free proposal today, or read our other PPC case studies below:
0 Comments